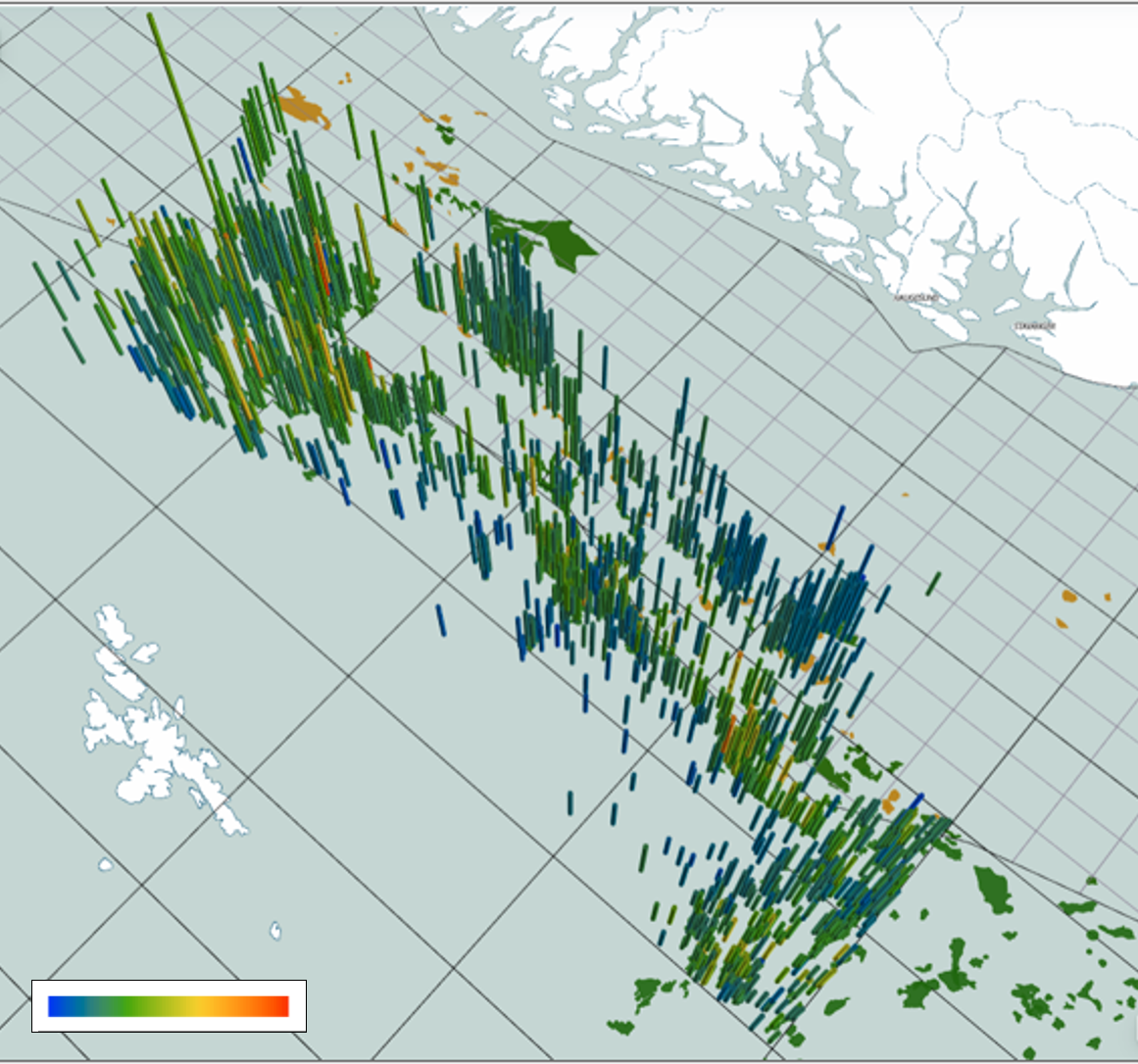
AI at Scale: Modernising subsurface interpretation with EarthNET
A machine learning-powered workflow enabling geoscientists to analyse and interpret large, complex subsurface datasets efficiently
Using AI-driven workflows, EarthNET reinterpreted nearly 5,000 North Sea wells, revealing over 450 missed hydrocarbon pay zones. This study shows how machine learning can unlock new value from legacy data in one of the world’s most mature basins
Building the data foundation: Earthnet Data Lake
The project began by ingesting data into the EarthNET Data Lake, which served as the unified environment for managing and analysing the 5,000+ well dataset. Key inputs included:
- 30,000 km of basic logs (GR, RES, DEN, DT, NPHI)
- 995 formation tops, 155 km of labelled training data (porosity, Sw, lithology)
- Depth-indexed logs (~90 million measurements)
Before initiating machine learning workflows, comprehensive quality control and data-conditioning were carried out using EarthNET’s automated and semi-automated tools. This process involved identifying and flagging bad hole conditions using caliper readings, borehole size, and density log mismatches. Non-physical values were removed, and anomalous zones were detected through domain-specific pattern recognition techniques (Figure 2).
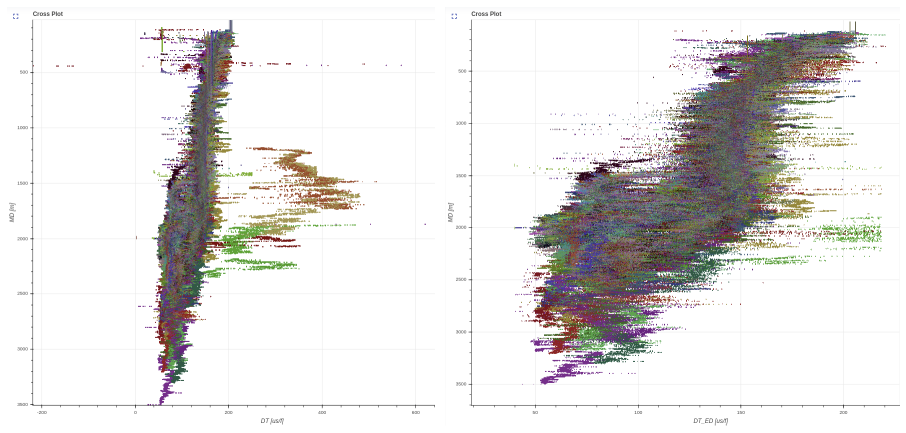
Predicting subsurface properties: AI wells
With a cleaned and contextualized database, the EARTHNET AI Wells module was used to predict rock and fluid properties for each well. The ML model was trained to generate porosity, water saturation (Sw), lithology, and pay flag (based on both static and dynamic classification).
These predictions were validated with blind QC on multiple well examples, showcasing predicted porosity against original logs and ground-truth labels. Pay classes were visualized across stratigraphic intervals with net reservoir and pay intervals filtered for wellbore condition, highlighting potential pay zones even in wells previously classified as dry. Well 35/11-13 (Figure 3), made the Byrding discovery in 2005, in the Heather Formation, high porosity and low water saturation were recorded in the Heather Formation, but interestingly also in the Draupne Formation.
Our workflow revealed a significant and much thicker oil accumulation in the segment adjacent to the well location. This was verified after our workflow by the use of the 2017 sidetracked well, which proved a 20 m oil-filled intra Draupner Fm, demonstrating the value of the workflow in identifying missed pay opportunities.
Seismic-scale analysis: AI seismic interpretation & properties
Once well-scale predictions were complete, attention shifted to the seismic domain using AI Seismic Interpretation and AI Seismic Properties.
A prime example is the Troll Field (Figure 1a and Figure 1b), located in the Norwegian Continental Shelf, where EarthNET’s seismic workflow demonstrated the full integration of AI-derived well and seismic data. This seismic-to-well integration showcases EarthNET’s ability to move beyond simple structure mapping and into full reservoir property prediction across volumes, enabling better prospect identification and risk mitigation. This combined approach enables rapid evaluation of large datasets.
One of the most powerful applications of this workflow was the identification of missed pay in historically drilled wells, many of which had been classified as dry.
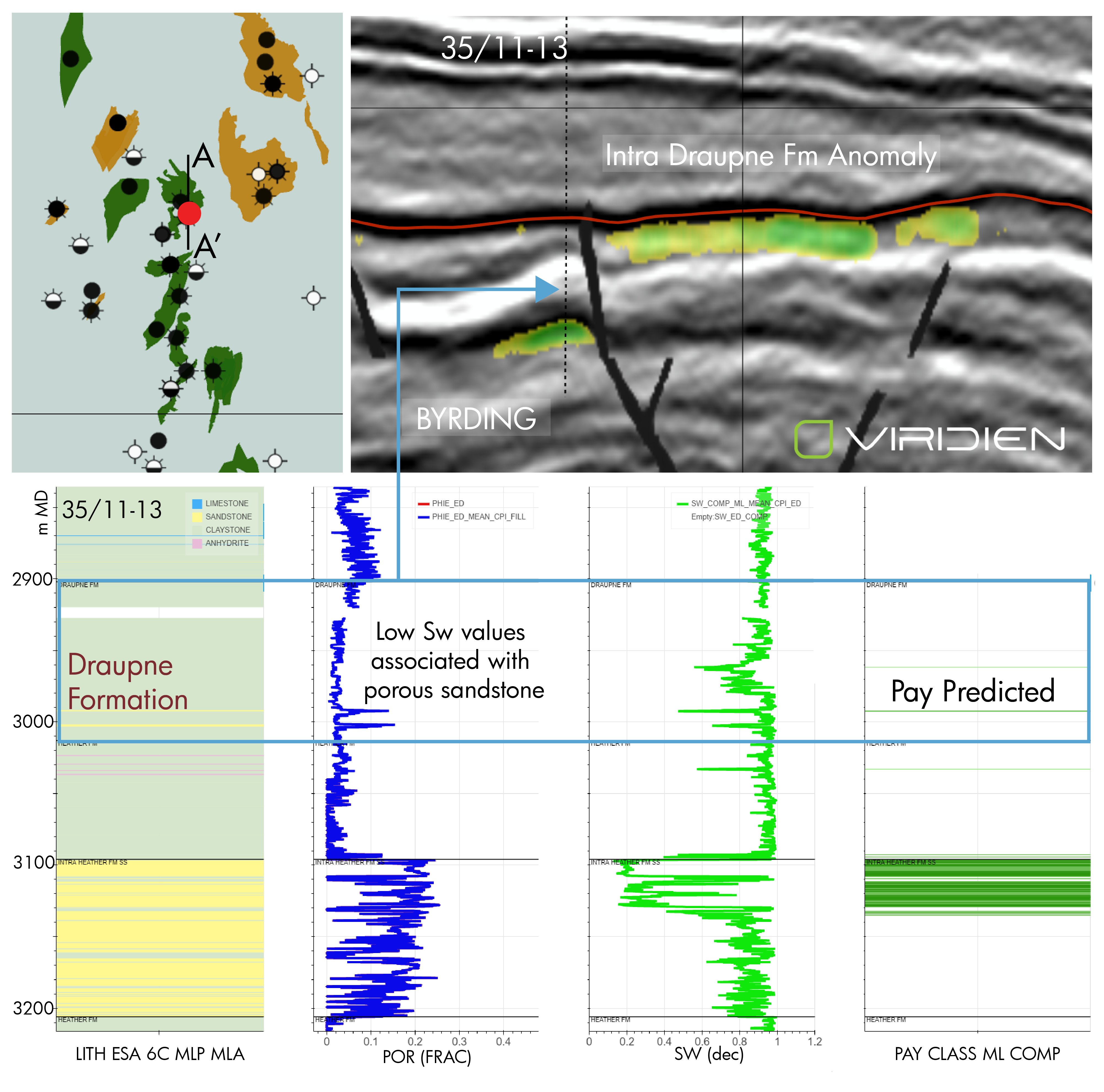
Real-world impact: Missed pay analysis
EarthNET’s ML models re-evaluated legacy logs and successfully identified 450+ new hydrocarbon pay intervals.
Application in mature fields: The Brent Field is characterized by a westerly dipping tilted fault block structure, forming a fault-controlled unconformity trap. The field comprises two primary reservoir units: Brent Group (Middle Jurassic) and Statfjord Formation (Lower Jurassic to Triassic). As expected, pay flags are observed (Figure 4) in the Brent and Statfjord groups. However, there is also a distinct drop in water saturation that aligns with a porosity spike within the Cormorant Formation, highlighted in the blue rectangle, suggesting a potential overlooked pay.
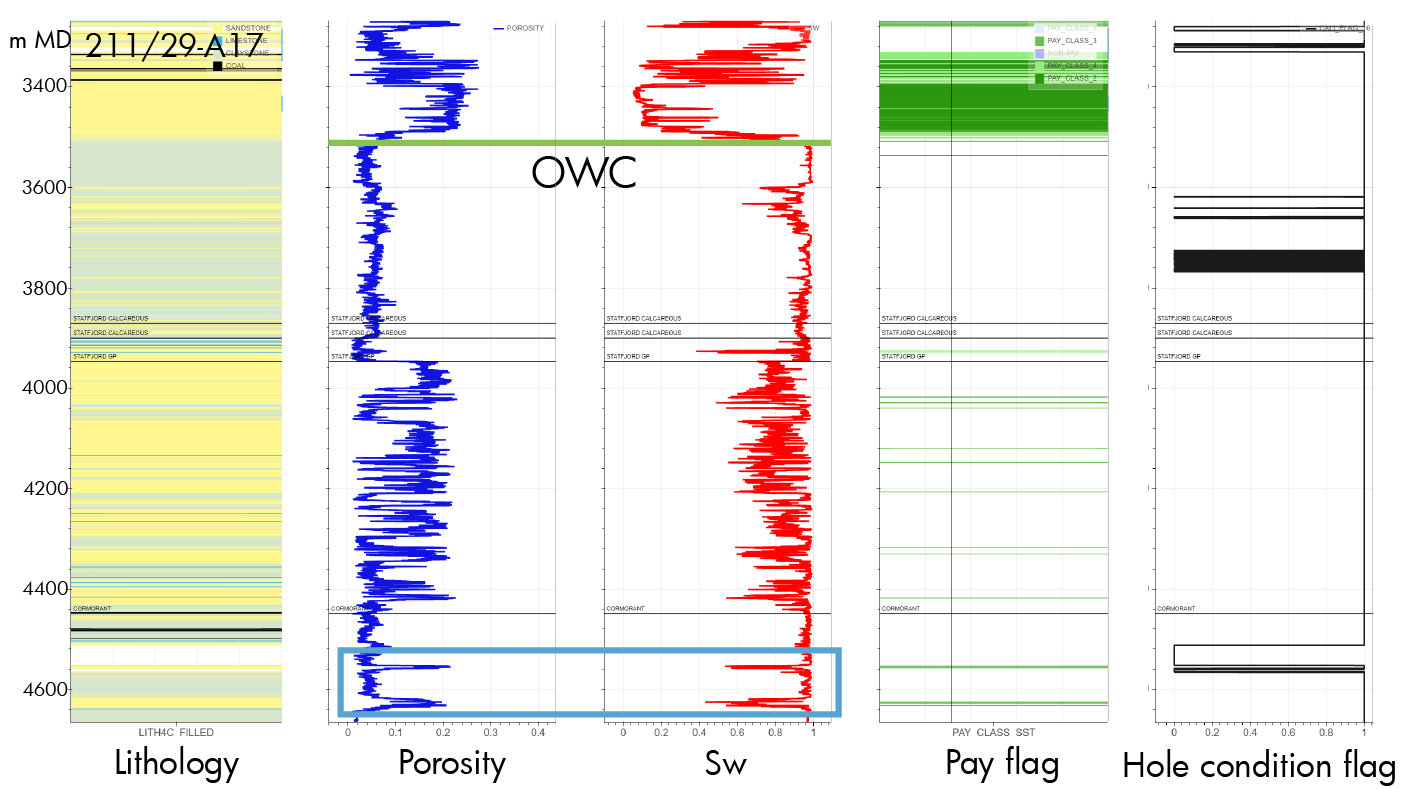
Viewer and insights: From prediction to decision support
Utilising EarthNet Insight, we conducted an evaluation of predictions and interpretations across the 5,000 wells, we interactively explored hydrocarbon pay by depth and formation, viewed lithology and porosity overlays, interpreted structural elements from seismic data, and analysed 3D reservoir property cubes.
This is illustrated in Figure 5, where true vertical depth (TVD) in meters is plotted against hydrocarbon pay across multiple wells. Notably, the oil-water contact (OWC) in the Brent Group is visible at approximately 2,800 m, while the Statfjord Group OWC appears around 3,000 m. When presented in this format, the observation from well 211/29-A17 stands out not just as an outlier, but as a potentially missed pay opportunity on a regional basis within the Cormmorant Formation. Additional candidate wells that show similar characteristics include 211/29-5 and 211/29-9.
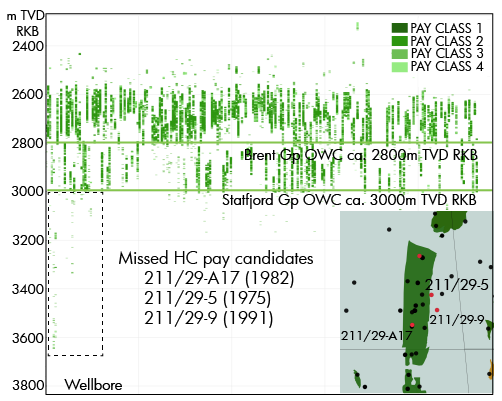
Conclusion
This North Sea case study illustrates how EarthNET is not just a collection of tools, but a digital ecosystem for subsurface analysis. By fully integrating seismic and well data, applying AI/ML-driven predictions, and enabling exploration geoscientists to visualize and validate in real time, the platform unlocks overlooked value in one of the world’s most data-rich basins. From missed pay reclassification to seismic-scale property prediction,
EarthNET has predicted pay intervales for ca 5,000 wells and identified more than 450 hydrocarbon pay intervals in wells registered as ‘dry’. This is reshaping how we as geoscientists interpret the subsurface – turning legacy data into tomorrow’s opportunities.