Unfortunately, diligent and scientific handling of subsurface uncertainty is rarely undertaken. Hesitance to embrace uncertainty often derives from time, data and budget constraints, limited interdisciplinary understanding, accompanied by claims of ‘black box’ algorithms and software solutions. Nevertheless, failing to address uncertainty impacts not only model accuracy and performance but can also culminate in the loss of valuable time and resources.
Traditionally, management decisions hinge on a deterministic (base-case) three-dimensional numerical representation of the subsurface. This model encompasses the best knowledge of all subsurface disciplines calibrated to the actual production response through history matching.
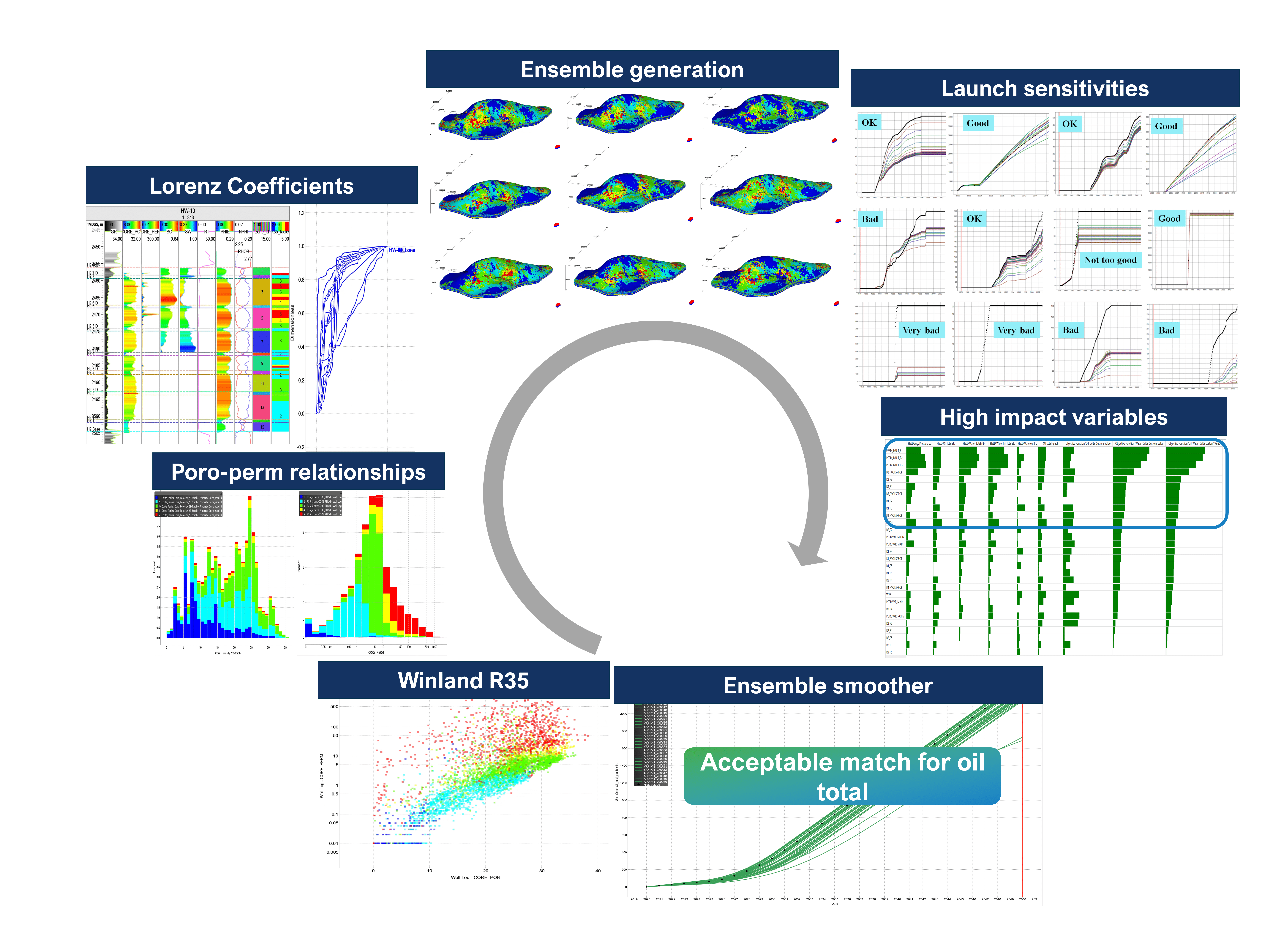
However, subsequent model modifications to approximate geological heterogeneity, accompanied by high and low case sensitivities, enter a trial-and-error loop to match the reservoir model to dynamic data. This phase invariably results in an underrepresentation of subsurface uncertainty.
Such approaches are no longer valid for complex, marginal and new energy assets under the current volatile economic climate, where uncertainties associated with projects and assets need to be identified, quantified, mitigated, and clearly communicated to decision makers.
Rock Flow Dynamics have deployed an ensemble-based approach inside of their tNavigator suite to manage uncertainty. The intuitive approach promotes the seamless integration of uncertainties of geological and reservoir parameters across all subsurface disciplines.
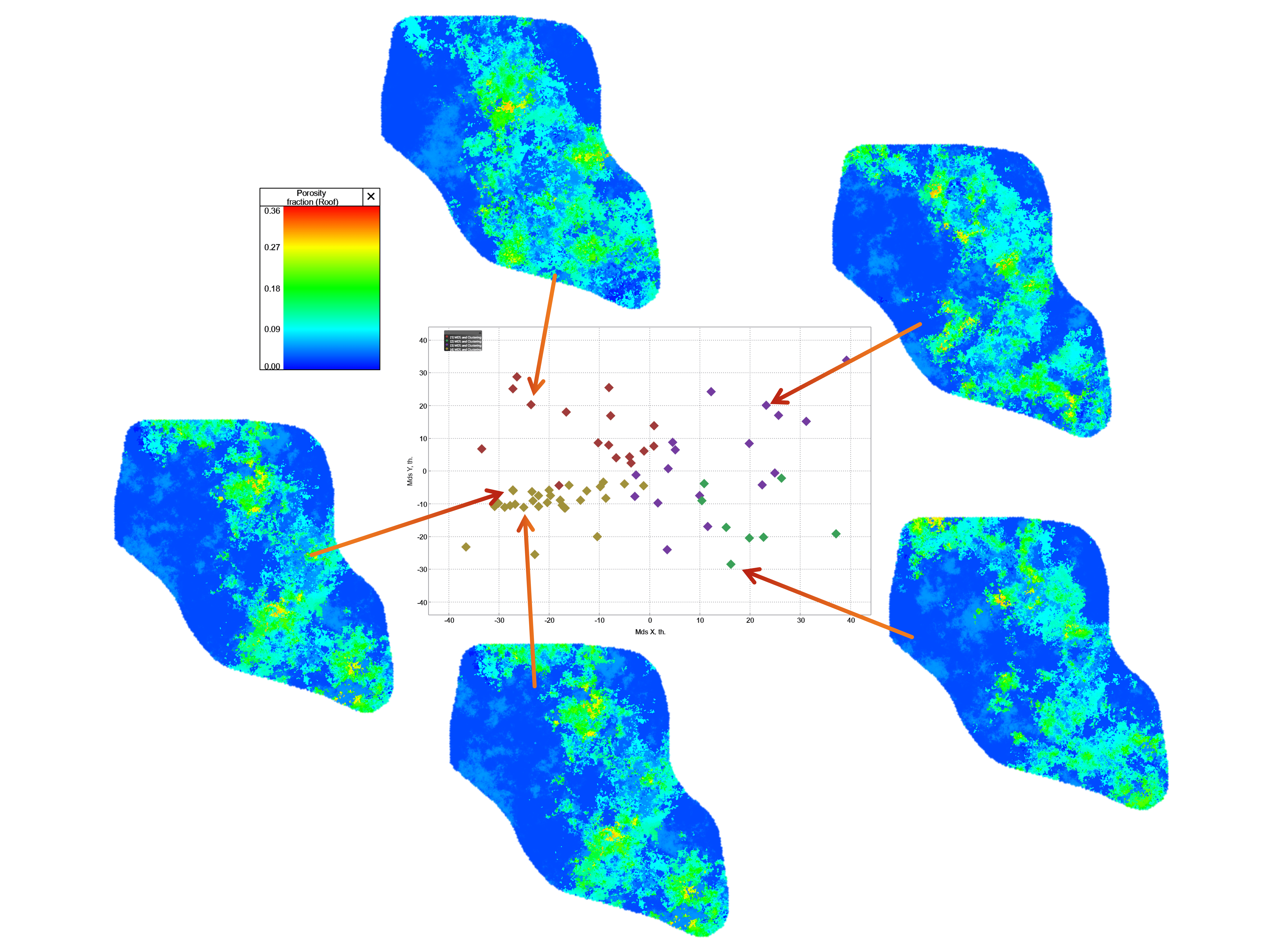
Exploring the uncertainty envelope
Ensemble-based modelling offers a method to generate a large range of initial reservoir models, an ensemble, that covers an array of possible static reservoir properties. Dynamic information can be seamlessly integrated to create a variety of probabilistic forecasts. In this manner, more time can be dedicated to understanding the reservoir and its inherent uncertainties, rather than building and forcing the single base case model to match historic production. This enables model diversity and widens the range for possible forecast scenarios that honor the input data; ultimately facilitating more informed reservoir management decisions.
One significant challenge in this context revolves around effectively managing a suite of reservoir models. The sheer volume of models makes it impractical to meticulously assess each one in isolation and conduct thorough comparisons with the rest. However, the concept of integration holds paramount importance. This applies not only to the seamless integration of geological, reservoir engineering, and surface-related elements, but extends to minimising barriers that hinder a comprehensive setup and analysis of simulation and modeling outcomes. Through the utilisation of uncertainty analysis and machine learning tools such as unsupervised dimension reduction and clustering techniques, this setup permits an easy overview of the reservoir model ensemble.
Harnessing new approaches
In the following section, we provide a concise overview of an integrated workflow. Rebuilding the COSTA model, an open-source synthetic carbonate case study based on the prolific Rub Al Khali Basin, UAE, a fully integrated workflow encompassing both static and dynamic uncertainties including facies, petrophysics and Corey exponents for oil and water relative permeabilities was developed.
First, a Latin Hypercube experiment, a statistical technique that efficiently samples multi-dimensional parameter spaces, was run to generate an initial ensemble of models. It permits an adequate coverage of subsurface uncertainties with a limited amount of generated reservoir models.
With the help of unsupervised dimension reduction techniques, such as Multi-Dimensional Scaling (MDS), the ensemble of models can be projected into a lower dimensional parameter space, where each model is represented by a single point (Figure 2). Points that are situated closely together represent models of greater similarity.
To optimise model selection while maintaining model diversity, it is sensible to only take models forward that are far apart from each other, i.e. the ones that are more dissimilar. This step can also be assisted through various unsupervised clustering or grouping algorithms, such as k-means clustering, to group together similar models and select a representative model of each cluster.
The initial ensemble was subsequently taken forward into an ensemble Kalman smoother to iteratively update the models to match the historical production data. Eventually, this leads to an updated ensemble that represents a weighted blend of existing models with a better match to the historic production data (Figure 1). It should be noted that the aforementioned dimension reduction and clustering techniques could now again be applied to the ensemble of matching models to ensure that a sensible tradeoff between maximising model diversity and simulation runtime is taken forward into the forecasting step.
Delivering value
Ensemble-based methods offer a novel way to explore uncertainties typified in the complex, more marginal and new energy assets that the industry now increasingly deals with. Fully identifying, exploring, and mitigating these uncertainties is crucial for informing successful asset management decisions. Ensemble-based modelling is fully integrated and spans a range of technical subsurface disciplines.
Due to easy parameterisation, ensemble runs can be generated throughout the lifespan of the asset and can be readily updated when new information is available. Moreover, they permit greater understanding of the reservoir as significant time is saved, rather than invested in matching large and unwieldy base case models that do not capture the full range of uncertainty in the subsurface.
This article was published in the latest issue of the GEO ExPro magazine. Please download the PDF of the magazine here.