The objective of the project, which will presented by Petter Dischington from NPD during the #DIGEX2021 conference next month, was to investigate prospectivity in the Paleocene Torsk Formation in the vicinity of the Alta and Gotha discoveries in the Norwegian Barents Sea.
The results of this work will be particularly interesting to learn about as Lundin Energy just reported a dry well in this area, with the reservoir being interpreted as having poor quality.
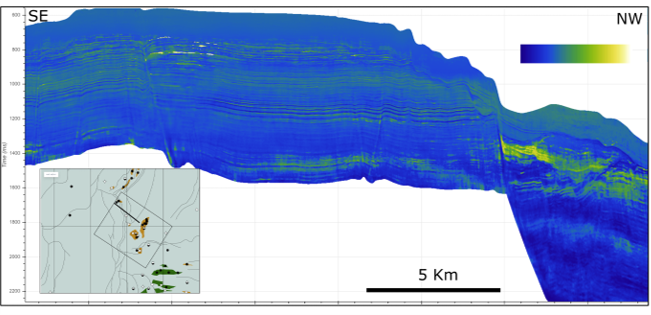
Initially, a team from Earth Science Analytics and the Norwegian Petroleum Directorate used 172 wells to build a supervised machine learning model for predicting rock and fluid-properties (porosity, saturation, and multi class lithology), concluded by a geological sense check of results.
Once properly trained and validated, these models were used to predict reservoir properties in 3D as a function of inverted elastic volumes.
The modelling workflow in a nutshell
The log imputation step included prediction of shear sonic and density as functions of other logs such GR, sonic, resistivity and depth.
First, reservoir properties were predicted as functions of standard logs (GR, resistivity, compressional sonic, shear sonic, density, and neutron) (Figure 1a).
Secondly, reservoir properties were predicted as functions of elastic properties (acoustic impedance and Vp/Vs). These models were trained solely on well data and used regional well data from almost all exploration wells in the Barents Sea.
Lundin Energy and partners provided 3D seismic data and corresponding elastic volumes (AI, VpVs, and SI), which have been used to predict porosity, water saturation and multiclass lithology volumes in 3D.
The way forward
Experience gained from both the well-based and seismic based analysis can provide a good, and resource-efficient basis for the NPD’s resource assessment, on basin-scale as well as more in-depth analysis of focus areas.
Do you want to hear more concrete examples on how companies are embracing the possibilities within the emerging domain of digitalisation and machine learning? Attend #DIGEX 2021 – 17-18 February. You can register here.
EDITORIAL TEAM